"""Simple tutorial using code from the TensorFlow example for Regression.
Parag K. Mital, Jan. 2016"""
'Simple tutorial using code from the TensorFlow example for Regression.\n\nParag K. Mital, Jan. 2016'
%matplotlib inline
import tensorflow as tf
import tensorflow.examples.tutorials.mnist.input_data as input_data
import numpy as np
import matplotlib.pyplot as plt
mnist = input_data.read_data_sets('MNIST_data/', one_hot=True)
Extracting MNIST_data/train-images-idx3-ubyte.gz
Extracting MNIST_data/train-labels-idx1-ubyte.gz
Extracting MNIST_data/t10k-images-idx3-ubyte.gz
Extracting MNIST_data/t10k-labels-idx1-ubyte.gz
print(mnist.train.num_examples,
mnist.test.num_examples,
mnist.validation.num_examples)
(55000, 10000, 5000)
print(mnist.train.images.shape, mnist.train.labels.shape)
((55000, 784), (55000, 10))
print(np.min(mnist.train.images), np.max(mnist.train.images))
(0.0, 1.0)
plt.imshow(np.reshape(mnist.train.images[100, :], (28, 28)), cmap='gray')
<matplotlib.image.AxesImage at 0x7fc304bdf750>
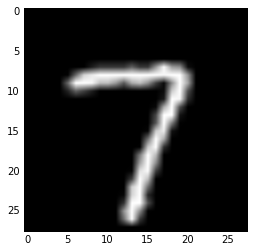
n_input = 784
n_output = 10
net_input = tf.placeholder(tf.float32, [None, n_input])
W = tf.Variable(tf.zeros([n_input, n_output]))
b = tf.Variable(tf.zeros([n_output]))
net_output = tf.nn.softmax(tf.matmul(net_input, W) + b)
y_true = tf.placeholder(tf.float32, [None, 10])
cross_entropy = -tf.reduce_sum(y_true * tf.log(net_output))
correct_prediction = tf.equal(
tf.argmax(net_output, 1), tf.argmax(y_true, 1))
accuracy = tf.reduce_mean(tf.cast(correct_prediction, "float"))
optimizer = tf.train.GradientDescentOptimizer(
0.01).minimize(cross_entropy)
sess = tf.Session()
sess.run(tf.initialize_all_variables())
batch_size = 100
n_epochs = 10
for epoch_i in range(n_epochs):
for batch_i in range(mnist.train.num_examples // batch_size):
batch_xs, batch_ys = mnist.train.next_batch(batch_size)
sess.run(optimizer, feed_dict={
net_input: batch_xs,
y_true: batch_ys
})
print(sess.run(accuracy,
feed_dict={
net_input: mnist.validation.images,
y_true: mnist.validation.labels
}))
0.8994
0.9236
0.9242
0.9152
0.9216
0.915
0.918
0.9256
0.926
0.9228
print(sess.run(accuracy,
feed_dict={
net_input: mnist.test.images,
y_true: mnist.test.labels
}))
0.923
"""
# We could do the same thing w/ Keras like so:
from keras.models import Sequential
model = Sequential()
from keras.layers.core import Dense, Activation
model.add(Dense(output_dim=10, input_dim=784, init='zero'))
model.add(Activation("softmax"))
from keras.optimizers import SGD
model.compile(loss='categorical_crossentropy',
optimizer=SGD(lr=learning_rate))
model.fit(mnist.train.images, mnist.train.labels, nb_epoch=n_epochs,
batch_size=batch_size, show_accuracy=True)
objective_score = model.evaluate(mnist.test.images, mnist.test.labels,
batch_size=100, show_accuracy=True)
"""
'\n# We could do the same thing w/ Keras like so:\nfrom keras.models import Sequential\nmodel = Sequential()\n\nfrom keras.layers.core import Dense, Activation\nmodel.add(Dense(output_dim=10, input_dim=784, init=\'zero\'))\nmodel.add(Activation("softmax"))\n\nfrom keras.optimizers import SGD\nmodel.compile(loss=\'categorical_crossentropy\', \n optimizer=SGD(lr=learning_rate))\n\nmodel.fit(mnist.train.images, mnist.train.labels, nb_epoch=n_epochs,\n batch_size=batch_size, show_accuracy=True)\n\nobjective_score = model.evaluate(mnist.test.images, mnist.test.labels,\n batch_size=100, show_accuracy=True)\n'