如何获取期权市场数据快照
来源:https://uqer.io/community/share/550274e4f9f06c7a9ae9a535
在本文中,我们将通过实际的市场的例子,展示如何在量化实验室中计算和展示期权的隐含波动率微笑。
import pandas as pd
from matplotlib import pylab
pd.options.display.float_format = '{:,>.4f}'.format
1. 获取市场数据
在本节中,我们使用数据API获取数据,并进行一些必要的数据转换。这里我们获取的是实时报价,是本 notebook 运行时的市场快照。
dataDate
交易日
dataTime
快照时间戳
optionId
期权代码
instrumentID
期权交易代码
contractType
期权类型,CO为看着,PO为看跌
strikePrice
行权价
expDate
到期日
lastPrice
最新价
optionSnapShot = OptionsDataSnapShot()
optionSnapShot[optionSnapShot.expDate == Date(2015,9,23)]
|
dataDate |
dataTime |
optionId |
instrumentID |
contractType |
strikePrice |
expDate |
lastPrice |
30 |
2015-03-13 |
13:24:12 |
10000031 |
510050C1509M02200 |
CO |
2.2000 |
September 23rd, 2015 |
0.3388 |
31 |
2015-03-13 |
13:24:17 |
10000032 |
510050C1509M02250 |
CO |
2.2500 |
September 23rd, 2015 |
0.3019 |
32 |
2015-03-13 |
13:24:22 |
10000033 |
510050C1509M02300 |
CO |
2.3000 |
September 23rd, 2015 |
0.2816 |
33 |
2015-03-13 |
13:24:27 |
10000034 |
510050C1509M02350 |
CO |
2.3500 |
September 23rd, 2015 |
0.2484 |
34 |
2015-03-13 |
13:24:32 |
10000035 |
510050C1509M02400 |
CO |
2.4000 |
September 23rd, 2015 |
0.2070 |
35 |
2015-03-13 |
13:24:36 |
10000036 |
510050P1509M02200 |
PO |
2.2000 |
September 23rd, 2015 |
0.0690 |
36 |
2015-03-13 |
13:24:41 |
10000037 |
510050P1509M02250 |
PO |
2.2500 |
September 23rd, 2015 |
0.0804 |
37 |
2015-03-13 |
13:24:47 |
10000038 |
510050P1509M02300 |
PO |
2.3000 |
September 23rd, 2015 |
0.0955 |
38 |
2015-03-13 |
13:24:52 |
10000039 |
510050P1509M02350 |
PO |
2.3500 |
September 23rd, 2015 |
0.1194 |
39 |
2015-03-13 |
13:24:58 |
10000040 |
510050P1509M02400 |
PO |
2.4000 |
September 23rd, 2015 |
0.1322 |
46 |
2015-03-13 |
13:24:52 |
10000047 |
510050C1509M02450 |
CO |
2.4500 |
September 23rd, 2015 |
0.1889 |
47 |
2015-03-13 |
13:24:58 |
10000048 |
510050P1509M02450 |
PO |
2.4500 |
September 23rd, 2015 |
0.1555 |
54 |
2015-03-13 |
13:24:32 |
10000055 |
510050C1509M02500 |
CO |
2.5000 |
September 23rd, 2015 |
0.1629 |
55 |
2015-03-13 |
13:24:36 |
10000056 |
510050P1509M02500 |
PO |
2.5000 |
September 23rd, 2015 |
0.1900 |
62 |
2015-03-13 |
13:24:32 |
10000063 |
510050C1509M02550 |
CO |
2.5500 |
September 23rd, 2015 |
0.1443 |
63 |
2015-03-13 |
13:24:36 |
10000064 |
510050P1509M02550 |
PO |
2.5500 |
September 23rd, 2015 |
0.2169 |
2. 计算隐含波动率以及相关Greeks
接着我们可以方便的使用内置函数 BSMImpliedVolatity 计算期权的隐含波动率。
price
市场报价或者模型价格
delta
期权价格关于标的价格的一阶导数
gamma
期权价格关于标的价格的二阶导数
rho
期权价格关于无风险利率的一阶导数
theta
期权价格关于到期时间的一阶导数(每日)
vega
期权价格关于波动率的一阶导数
analyticResult = OptionsAnalyticResult()
analyticResult.loc[:10, ['optionId', 'contractType', 'strikePrice', 'expDate', 'lastPrice', 'vol', 'delta', 'gamma', 'rho', 'theta', 'vega']]
|
optionId |
contractType |
strikePrice |
expDate |
lastPrice |
vol |
delta |
gamma |
rho |
theta |
vega |
1 |
10000002 |
CO |
2.2500 |
March 25th, 2015 |
0.2184 |
0.2259 |
0.9886 |
0.2947 |
0.0730 |
-0.0458 |
0.0133 |
2 |
10000003 |
CO |
2.3000 |
March 25th, 2015 |
0.1730 |
0.2867 |
0.9165 |
1.1965 |
0.0687 |
-0.2996 |
0.0687 |
3 |
10000004 |
CO |
2.3500 |
March 25th, 2015 |
0.1229 |
0.2177 |
0.8963 |
1.8495 |
0.0687 |
-0.2670 |
0.0806 |
4 |
10000005 |
CO |
2.4000 |
March 25th, 2015 |
0.0814 |
0.2166 |
0.7676 |
3.1504 |
0.0596 |
-0.4503 |
0.1367 |
8 |
10000009 |
PO |
2.3500 |
March 25th, 2015 |
0.0076 |
0.2482 |
-0.1332 |
1.9373 |
-0.0111 |
-0.3633 |
0.0963 |
9 |
10000010 |
PO |
2.4000 |
March 25th, 2015 |
0.0159 |
0.2346 |
-0.2488 |
3.0197 |
-0.0207 |
-0.5061 |
0.1419 |
10 |
10000011 |
CO |
2.2000 |
April 22nd, 2015 |
0.2778 |
0.2703 |
0.9081 |
0.7466 |
0.2152 |
-0.1661 |
0.1347 |
3. 构造波动率曲面
但是对于市场参与者而言,像刚才这样仅仅观察的线的结构不够。他们需要看到整个市场以到期时间,行权价为轴的波动率曲面(Volatility Surface)。除此之外,他们更想知道,波动率曲面上,那些并不是市场报价点的值,至少是个估计。这样的波动率曲面构造,往往需要依赖某种模型,或者某种插值方法。在这一节中,我们将介绍使用 CAL 中的波动率曲面构造函数。
以下的例子基于 CAL 函数: VolatilitySurfaceSnapShot
3.1 基于SABR模型的波动率曲面
volInterpolatorSABR = VolatilitySurfaceSnapShot(optionType = 'CALL', interpType = 'SABR')
volInterpolatorSABR.plotSurface(startStrike = 2.2,endStrike = 2.6)
volInterpolatorSABR.volalitltyProfileFromPeriods([2.2, 2.3, 2.4, 2.5, 2.6], ['1M', '2M', '3M', '6M', '9M'])
|
1M |
2M |
3M |
6M |
9M |
2.2000 |
0.2720 |
0.2406 |
0.2327 |
0.2531 |
0.2545 |
2.3000 |
0.2048 |
0.2207 |
0.2345 |
0.2546 |
0.2557 |
2.4000 |
0.2245 |
0.2341 |
0.2389 |
0.2525 |
0.2533 |
2.5000 |
0.2241 |
0.2328 |
0.2381 |
0.2479 |
0.2484 |
2.6000 |
0.2311 |
0.2356 |
0.2362 |
0.2425 |
0.2429 |
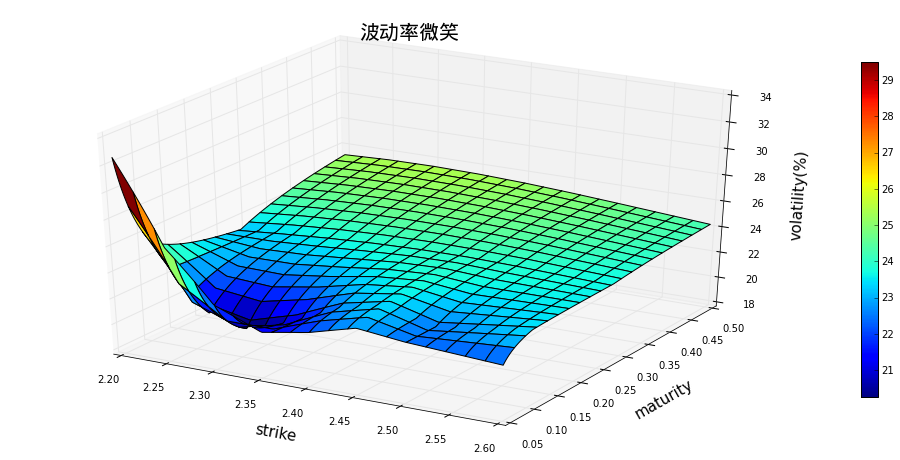
3.2 基于SVI模型的波动率曲面
volInterpolatorSVI = VolatilitySurfaceSnapShot(optionType = 'CALL', interpType = 'SVI')
volInterpolatorSVI.plotSurface(startStrike = 2.2,endStrike = 2.6)
volInterpolatorSVI.volalitltyProfileFromPeriods([2.2, 2.3, 2.4, 2.5, 2.6], ['1M', '2M', '3M', '6M', '9M'])
|
1M |
2M |
3M |
6M |
9M |
2.2000 |
0.2769 |
0.2476 |
0.2369 |
0.2566 |
0.2580 |
2.3000 |
0.2121 |
0.2223 |
0.2340 |
0.2535 |
0.2545 |
2.4000 |
0.2170 |
0.2292 |
0.2365 |
0.2504 |
0.2512 |
2.5000 |
0.2290 |
0.2357 |
0.2389 |
0.2474 |
0.2479 |
2.6000 |
0.2401 |
0.2417 |
0.2413 |
0.2508 |
0.2514 |

3.3 基于Balck波动率插值的波动率曲面
volInterpolatorVariance = VolatilitySurfaceSnapShot(optionType = 'CALL', interpType = 'BlackVariance')
volInterpolatorVariance.plotSurface(startStrike = 2.2,endStrike = 2.6)
volInterpolatorVariance.volalitltyProfileFromPeriods([2.2, 2.3, 2.4, 2.5, 2.6], ['1M', '2M', '3M', '6M', '9M'])
|
1M |
2M |
3M |
6M |
9M |
2.2000 |
0.2676 |
0.2380 |
0.2202 |
0.2516 |
0.2537 |
2.3000 |
0.2082 |
0.2270 |
0.2441 |
0.2660 |
0.2672 |
2.4000 |
0.2277 |
0.2325 |
0.2341 |
0.2404 |
0.2408 |
2.5000 |
0.2278 |
0.2363 |
0.2408 |
0.2463 |
0.2466 |
2.6000 |
0.2252 |
0.2324 |
0.2365 |
0.2517 |
0.2526 |

4. 组合计算
在本节中,我们假设客户已经拥有了自己的期权头寸,希望利用量化实验室的功能进行风险监控。我们假设有以下的期权头寸:
期权代码 |
数量 |
行权价(¥) |
到期时间 |
10000004 |
-7000 |
2.35 |
2015-03-25 |
10000011 |
2000 |
2.20 |
2015-04-22 |
10000027 |
5000 |
2.25 |
2015-06-24 |
10000047 |
3000 |
2.45 |
2015-09-23 |
然后我们构造 OptionBook
:
optionIDs = ['10000011', '10000027', '10000004', '10000047']
amounts = [2000, 5000, -7000, 3000]
optBook = OptionBook(optionIDs, amounts)
print u'期权头寸:'
optBook.description()
期权头寸:
|
dataDate |
dataTime |
optionId |
instrumentID |
contractType |
strikePrice |
expDate |
lastPrice |
amount |
0 |
2015-03-13 |
13:24:58 |
10000004 |
510050C1503M02350 |
CO |
2.3500 |
March 25th, 2015 |
0.1229 |
-7000 |
1 |
2015-03-13 |
13:24:32 |
10000011 |
510050C1504M02200 |
CO |
2.2000 |
April 22nd, 2015 |
0.2778 |
2000 |
2 |
2015-03-13 |
13:24:52 |
10000027 |
510050P1506M02250 |
PO |
2.2500 |
June 24th, 2015 |
0.0450 |
5000 |
3 |
2015-03-13 |
13:24:52 |
10000047 |
510050C1509M02450 |
CO |
2.4500 |
September 23rd, 2015 |
0.1889 |
3000 |
4.1 使用Black插值模型计算组合风险
optBook.riskReport(volInterpolatorVariance)
|
optionId |
vol |
price |
delta |
gamma |
rho |
theta |
vega |
0 |
10000004 |
0.2060 |
-860.3000 |
-6370.8417 |
-12316.8687 |
-488.8540 |
1592.3418 |
-508.3851 |
1 |
10000011 |
0.2634 |
555.6000 |
1828.2807 |
1456.7054 |
433.8000 |
-307.9681 |
256.2961 |
2 |
10000027 |
0.2484 |
220.5000 |
-1103.5286 |
4552.0335 |
-831.0864 |
-856.2742 |
1945.3136 |
3 |
10000047 |
0.2509 |
566.7000 |
1659.5347 |
2626.2983 |
1876.5865 |
-503.9843 |
2135.1356 |
portfolio |
NaN |
nan |
482.5000 |
-3986.5549 |
-3681.8315 |
990.4461 |
-75.8848 |
3828.3602 |
4.2 使用SABR模型组合风险
optBook.riskReport(volInterpolatorSABR)
|
optionId |
vol |
price |
delta |
gamma |
rho |
theta |
vega |
0 |
10000004 |
0.2157 |
-865.4365 |
-6301.5462 |
-12703.7735 |
-483.0602 |
1800.8937 |
-549.0791 |
1 |
10000011 |
0.2523 |
552.8686 |
1845.2432 |
1405.9255 |
438.6890 |
-272.7679 |
236.9632 |
2 |
10000027 |
0.2347 |
194.1368 |
-1048.6009 |
4677.9921 |
-785.3771 |
-785.2668 |
1888.5079 |
3 |
10000047 |
0.2511 |
566.9933 |
1659.5667 |
2624.8517 |
1876.4726 |
-504.2584 |
2135.1279 |
portfolio |
NaN |
nan |
448.5622 |
-3845.3372 |
-3995.0043 |
1046.7243 |
238.6007 |
3711.5199 |
4.3 使用SVI模型组合风险
optBook.riskReport(volInterpolatorSVI)
|
optionId |
vol |
price |
delta |
gamma |
rho |
theta |
vega |
0 |
10000004 |
0.2126 |
-863.7639 |
-6323.4081 |
-12591.1718 |
-484.8898 |
1734.2876 |
-536.4362 |
1 |
10000011 |
0.2634 |
555.6000 |
1828.2807 |
1456.7054 |
433.8000 |
-307.9681 |
256.2961 |
2 |
10000027 |
0.2355 |
195.6318 |
-1051.9049 |
4670.9045 |
-788.1010 |
-789.3710 |
1892.0017 |
3 |
10000047 |
0.2495 |
563.6855 |
1659.2077 |
2641.2567 |
1877.7596 |
-501.1669 |
2135.2142 |
portfolio |
NaN |
nan |
451.1534 |
-3887.8246 |
-3822.3052 |
1038.5689 |
135.7815 |
3747.0758 |
5 比较不同模型的拟合市场数据的能力
这里我们比较不同的模型,对于市场数据的拟合能力。这里我们可以观察到单论你和能力 BlackVarianceSurface > SviCalibratedVolSruface > SABRCalibratedVolSruface
。这里我们并不想下这样的结论:这些模型的优劣也有相同的排序。
另一个我们可以观察到的现象,对于近月合约(流动性最好),波动率微笑是最规则的。在这个期限上,三种模型的拟合都很到位。随着期限的上升,流动性的下降,买卖价差也随之扩大。这时候波动率微笑变得愈发不规则,这个时候一个完美拟合至市场的模型是否必要,是一个很大的问题:如果市场报价并不理性,一个优秀的模型应该可以指出这种不合理点,而不是简单的接受市场的非理性。
from matplotlib import pylab
strikes = sorted(analyticResult['strikePrice'].unique())
expiries = [Date(2015,3,25),Date(2015,4,25),Date(2015,6,25),Date(2015,9,25)]
maturity = [(date - EvaluationDate())/ 365.0 for date in expiries]
volSurfaces = [volInterpolatorSABR, volInterpolatorSVI]
def plotModelFitting(index, volSurfaces, legends = ['Market Quote', 'SABR', 'SVI']):
data = volInterpolatorVariance.volatility(strikes, maturity[index], True)
pylab.plot(strikes, data, 'r+-.', markersize = 8)
for s in volSurfaces:
data = s.volatility(strikes, maturity[index], True)
pylab.plot(strikes, data)
pylab.xlabel('Strike')
pylab.ylabel('Volatility')
pylab.legend(legends, loc = 'best', fontsize = 12)
pylab.title(u'行权结算日: ' + str(expiries[index]), fontproperties = font, fontsize = 20)
pylab.grid(True)
pylab.subplots(2,2, figsize = (16,14))
for i in range(1,5):
pylab.subplot('22' + str(i))
plotModelFitting(i-1, volSurfaces)
